Play/Pause
Video does not contain audio
A Degree That Matters
At WPI, you'll use your unmatched college experience—built around STEM, business, global engagement, and the humanities—to work in teams here at home and around the world to create solutions to problems faced by real people.
Explore the possibilities with more than 70 undergraduate and graduate degree programs across four schools: Engineering, Arts & Sciences, Business, and Global.
FIND A DEGREE PROGRAM:
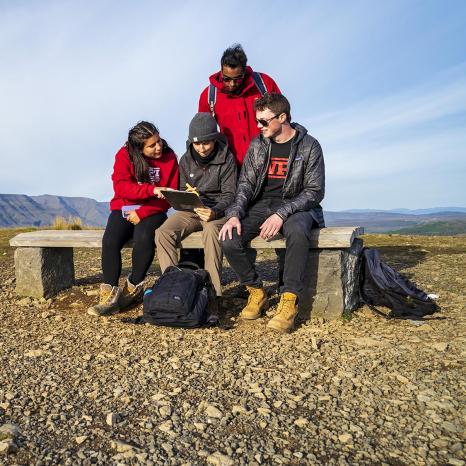
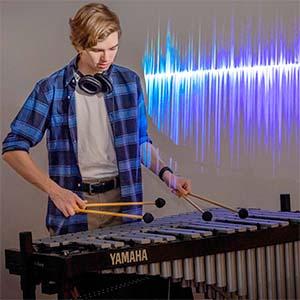
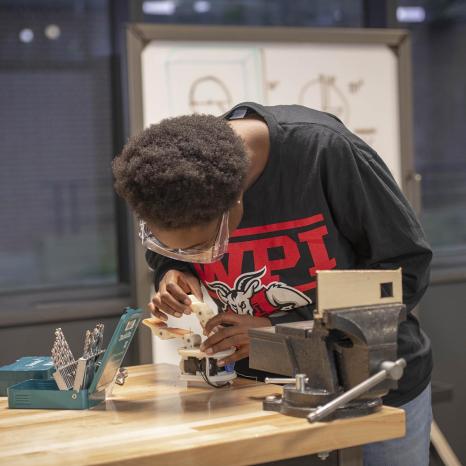
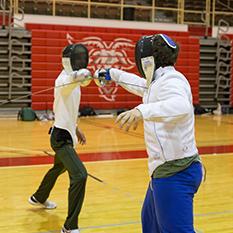
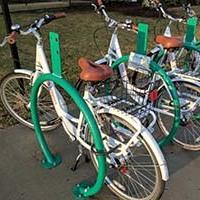

Features from the WPI Journal
See how DraftKings co-founder Paul Liberman ’05 built a gaming empire with an engineering mindset; why Gabriela Hoops ’19 was closely watching last summer’s 2023 Women’s World Cup in Australia; and how Professor Jamal Yagoobi is working with industry to address energy-hogging drying technology on the manufacturing floor. In addition, read about adaptive golfer Doug Shirakura ’24 and his quest to inspire; and the plot twist that had Nina Simon ’03 pivoting from global nonprofit CEO to bestselling mystery author.