TRIAD Grants Awarded 2019-20
To encourage multidisciplinary collaborative research and to attract external funding to the university’s research, WPI launched the Transformative Research and Innovation, Accelerating Discovery (TRIAD) seed grant program in the fall. A semi-random selection process was used, a process that ensured that funded projects meet the administrative requirements of the program and guarantees portfolio diversity. We have now funded 17 projects, all promising to be exciting innovative collaborations with faculty from all academic departments.
TRIAD Grants Will Expand Multidisciplinary Research Possibilities
Read this Herd story to find out more about how the TRIAD seed grant program started and how it helps advance WPI research.
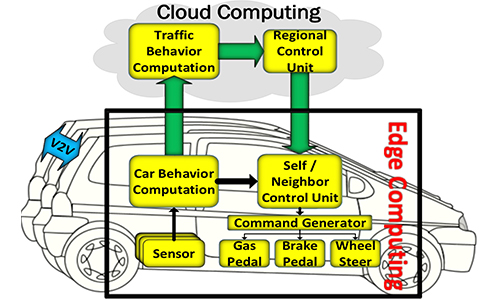
A Journey from High Dimensional PDE to Reinforcement Learning and Its Applications
Principal Investigator: Qingshuo Song
Collaborators: Yanhua Li, Seyed A Zekavat
5:30 PM
Dinner at the dining hall—our team usually ate together and discussed the project if needed.
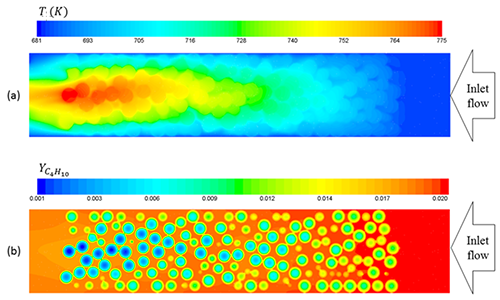
Chemical Processing and Machine Learning
Principal Investigator: Randy C Paffenroth
Collaborators: Anthony G Dixon, Michael T Timko
8:30 PM
Stop by a local ice cream shop for some dessert.
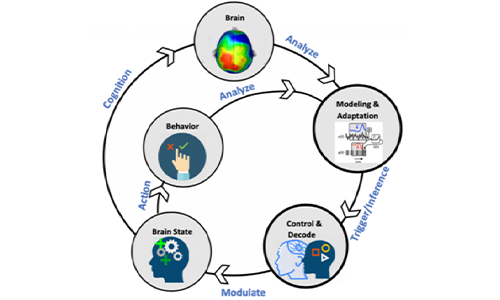
Closed-Loop BCI Using Adaptive Kinetic Architectural Design to Regulate Human Emotional States
Principal Investigator: Ali Yousefi
Collaborators: Mohamad Farzinmoghadam, Erin Solovey
8:00am
I make a to-do list of all the things I want to accomplish during the day.
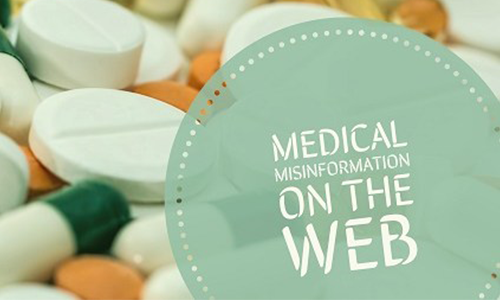
Combating Online Health Misinformation: An Integration of Behavioral and Computational Approaches
Principal Investigator: Nima Kordzadeh
Collaborators: Kyumin Lee, Brenton Faber
9:30 AM
Partake in tourist activities (hiking, swimming, boat rides, castle tours, etc.).

Complex Simulations for Local Environmental Policy: Digitization, Analysis, and Activism
Principal Investigator: Shamsnaz Virani Bhada
Collaborators: Constance Clark, Jennifer deWinter, Paul P Mathisen
10:00 PM
Go back to inn and hang out with everyone.

Creating a Digital Platform for Understanding Fatigue in Lymphangioleiomyomatosis Patients
Principal Investigator: Ulkuhan Guler
Collaborators: Pratap M Rao, Bengisu Tulu
7:30 AM
Wake up and get ready for breakfast.
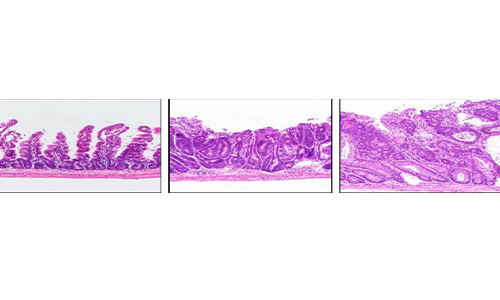
Detection of Bioelectrical Changes as a Function of Neoplastic Progression
Principal Investigator: Ahmet C Sabuncu
Collaborators: Izabela R Stroe, Cagdas D Onal, Masqsood Ali Mughal
Read the description
Early detection is the hallmark of successful cancer treatment. However, many tumors remain clinically occult until they are far advanced. Carcinoma is one of the most common types of cancer that proliferates in the epithelial tissues, with colorectal carcinoma cancer (CRC) being the 3rd most common cancer in males and 2nd in females, and globally the 4th cause for cancer death worldwide. New research shows that the incidence of CRC is increasing among younger adults for reasons that are not yet known. It is predicted that by 2030, CRC incidence rates will increase by 90% for people aged 20 to 34 years and by 28% for people aged 35 to 49 years.
Endoscopy is the gold standard for CRC detection and removing of carcinoma and its premalignant precursor, dysplasia. However, its accuracy is suboptimal with 41% of precancerous adenomas are missed during examination. This is mostly due to: (1) the difficulty of identification of carcinoma in patients with preexisting conditions (such as inflammatory bowel disease, or in patients who have been previously biopsied), and (2) the inherent limitations of the technique that does not allow a full visualization of early carcinoma in a background of dysplasia and hidden polyps especially the ones behind colonic folds and flexures.
We propose to develop a novel diagnostic tool for endoscopy that improves the detection accuracy of colorectal cancer and therefore increases the survival rate of cancer patients. Our proposed endoscope will incorporate an impedance spectroscope that scans the bioelectrical properties of tissues, such as tissue capacitance and conductance. It will enable a real-time bioelectric fingerprint of the colon for early detection of colorectal cancer, while minimally impacting the standard colonoscopy procedure.
In this pilot, we will: (1) investigate the changes in the bioelectrical parameters of normal, precancerous and cancerous cells and tissues, (2) develop computational models to analyze and decompose impedance spectra into biologically relevant parameters of tissues, namely, cell membrane capacitance, transmembrane and extracellular conductance, and cytoplasm conductance. Correlations will be sought between these parameters and the severity of carcinoma. Results will be useful in formulating a hypothesis for the new endoscope design.
These preliminary results will be used for future proposals to NIH and similar funding agencies.

Does Mechanical Stress Facilitate Muscle-Neuron Integration?
Principal Investigator: Suzanne F Scarlata
Collaborators: Kristen Billiar, Sarah D Olson, George D. Pins
11:00am
Spend the day working in the lab with different plants and cell cultures
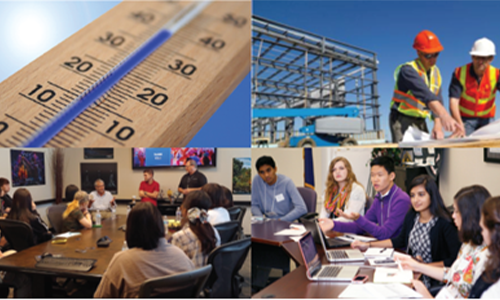
Environmental Stressors and Decision-making Performance in the Context of Climate Change
Principal Investigator: Shichao Liu
Collaborators: Soussan Djamasbi, Gbetonmasse Blaise Somasse, Sarah Strauss
7:00pm
After dinner and meetings, the rest of the day is spent catching up on homework, relaxing, and spending time with friends and family.
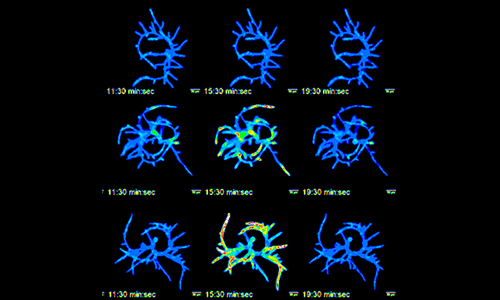
How Do Plants Sense Pathogens? Molecular Receptors and Cellular Signaling
Read the description
Over millions of years, plants have coexisted and coevolved with microorganisms. To survive and adapt, all plants have to be able to identify microbes and determine if they are pathogenic, beneficial, or neutral. The mechanisms for detection are complex and can be neutralized by pathogenic organisms. A highly-conserved mechanism that plants use to detect fungi comprises membrane receptors to fungal cell wall components; the most investigated of these is the receptor for chitin oligosaccharides. When the chitin receptor is activated, the signal is transduced by a rapid increase in cytosolic calcium --by a process similar to what takes place in neuronal and muscle cells. Downstream from this calcium increase, the signaling process results in changes in gene expression, including defense-related genes. The chitin response is conserved in all land plants, including the powerful model moss, Physcomitrella patens. We have generated transgenic P. patens plants expressing the cytosolic calcium fluorescent sensor, GCaMpf6. We found that soon after chitin treatment, the plants stop growing and produce oscillatory calcium spiking. We are currently analyzing the significance of these oscillations by evaluating the hypothesis that their frequency and amplitude encode information that is interpreted by downstream mechanisms. To fully develop this project, we need to assemble an interdisciplinary team with expertise in advanced three-dimensional microscopy, signal/noise processing, and mathematical modeling. It is anticipated that this project will provide sufficient preliminary results to submit a collaborative grant proposal to the NSF/USDA program on Plant Biotic Interactions. https://www.nsf.gov/pubs/2018/nsf18590/nsf18590.htm

ICME Approach to Design Novel Safe Lithium Electrode
Principal Investigator: James L Urban
Collaborators: Yu Zhong, Albert Simeoni, Milosh T Puchovsky, Winston Soboyejo
1:00 PM
Head out to lunch either nearby or further in the city.

Multi-scale Mechanical Characterization and Modeling of the Brain for Injury Prevention
Principal Investigator: Songbai Ji
Collaborators: Yuxiang Liu, Yihao Zheng
10:30 AM
Spend the afternoon going on a hike in the park.
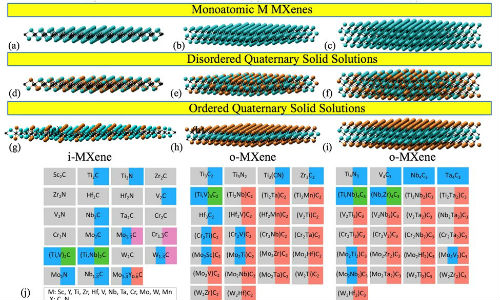
MXenes: New 2D Materials for Applications in Catalysis, Sensing and Electronics
Principal Investigator: Lyubov Titova
Collaborators: Douglas T Petkie, Andrew R Teixeira, Aaron Deskins
8:00am
My day starts with a stop at the Campus Center to get coffee and a bagel to eat during class.

Photoacoustic Imaging of Neurovascular Bundles for Robot-Assisted Laparoscopic Pelvic Surgery
Principal Investigator: Haichong Zhang
Collaborators: Loris Fichera, Gregory S Fischer, Benjamin Nephew
Read the description
The objective of this proposal is to explore the creation of a novel spectroscopic photoacoustic (PA) imaging solution to detect neurovascular bundles (NVBs) during robot-assisted pelvic surgical procedures including radical prostatectomies. This research is motivated by the high rate of nerve damage associated post-operative complications from pelvic surgery and the need for a reliable guidance system to assist surgeons in detecting and preserving these delicate structures. Especially in the case of prostatectomy, accidental damage to these delicate structures is a leading cause of post-operative erectile dysfunction in prostate cancer patients. There is a strong demand in the medical community for a technology to intraoperatively detect the location of NVBs. These structures are embedded in soft tissue (i.e. the “fascia” layers surrounding the prostate), and are thus invisible from the surface, making their detection challenging. Recent advances in medical imaging technology, namely the ability to harness the PA effect to enable high optical contrast with ultrasonic spatial resolution in deep tissue, opens new possibilities for the visualization of NVBs. The specific objective of the proposed one-year effort is to provide proof of concept evidence for the spectroscopic PA detection of NVBs that will support the systematic integration of this technology in robot-assisted laparoscopic nerve-sparing pelvic surgery such as radical prostatectomy. To evaluate the feasibility of detecting NVBs via PA imaging, we initially propose to spectroscopically characterize types of biological tissue commonly encountered during prostate cancer surgery and will then investigate methods to separate the PA contrast of the NVB from that of surrounding tissue. Our research is driven by the following two hypotheses: 1) The PA contrast of nerves and blood vessels, i.e. the main constituents of NVBs, can be selectively decomposed from that of surrounding structures; 2) Nerves, blood vessels, and other types of soft tissue surrounding the prostate each present a unique absorption spectrum in the Near-Infrared (NIR) range of wavelengths (700-1400 nm). While the ultimate goal of our research is to develop a PA imaging instrument that can be integrated in the workflow of robot-assisted pelvic surgery, this proposal focuses on verifying the above-mentioned hypotheses. It will culminate in a proof-of-concept of PA-based NVB detection in an in vivo rat model.
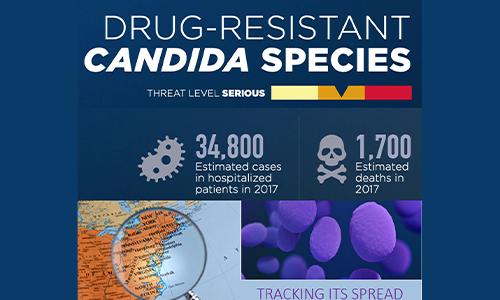
SUPER BUGS, Genomics-Driven Molecular and Epidemiological Modeling of Multidrug Resistance
Principal Investigator: Reeta Prusty Rao
Collaborators: Eric M Young, Jian Zou
10:00 AM
Attend weekly meeting with advisor to check in on progress (as our presentation day approached, we met with our advisor multiple times a week to ensure that our work would be as effective as possible).

Solid electrolyte with high ionic conductivity and stability through high throughput experiments, phase modeling and AI
Principal Investigator: Yan Wang
Collaborators: Yu Zhong, Xiangnan Kong
6:30 PM
Free time, usually spent either in the common room of my cottage playing games with housemates or taking the Island Explorer bus into Bar Harbor for a grocery run.

Zero- or Negative-Emissions Aerospace Fuel
Principal Investigator: Jagannath Jayachandran
Collaborators: Adam C Powell, Ronald Grimm
6:30 PM
Spend part of the evening playing card games.